France-BioImaging Challenge is back! Fuse My Cells
For a second running year, France-BioImaging organizes its data machine learning competition: we introduce you Fuse My Cells challenge!
What is the Challenge?
France-BioImaging‘s Fuse My Cells challenge aims to advance new methods for 3D image-to-image fusion using deep learning in the fields of biology and microscopy.
The main objective of the Fuse My Cells challenge is to predict a fused 3D image using only one or two available 3D views, providing a practical solution to the limitations of current microscopy techniques, such as improving image quality, extending the duration of live imaging, saving on the photon budget, and facilitating image analysis.
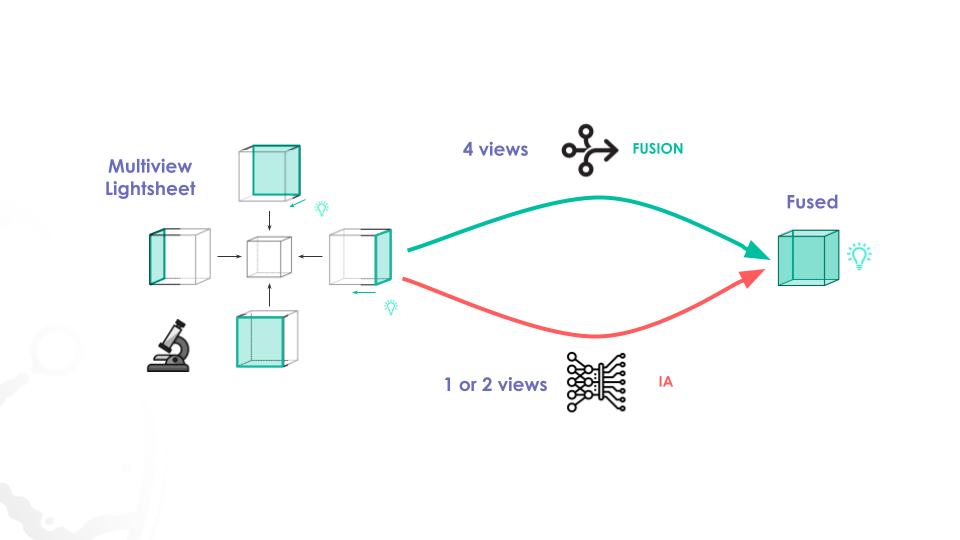
More information about the challenge: https://fusemycells.grand-challenge.org/fusemycells/
How to participate?
As last year Challenge, the competition is divided in two phases:
- Test phase (on three 3D images) to familiarize with the algorithm submission procedure, with the possibility to have five submissions (not taken into account in the final ranking)
- Evaluation phase (on thirsty 3D images) with a single submission to obtain the final ranking. Algorithms must be tested in the Test phase and will not be tested in this phase.
So, you have until the end of the first phase, on February 28, 2025, to register and participate at this Fuse My Cells challenge. Nonetheless, you can start working on your preliminary algorithm and tests on January 31st, 2025 (with the release of the training database)!
What are the prizes?
For top 3 winners:
- Award certificate
- A challenge paper will be written with the organizing team’s members for submission to journals
- Invitation to publish their methods in the proceedings of the IEEE International Symposium on Biomedical Imaging 2025s
- Support and integration of open source code into open science image processing and analysis software (e.g. BioImage Model Zoo, Napari)
More information to come.
Why launching a challenge?
If successful, the Fuse My Cells challenge can lead to robust methods for image deep learning fusion processing. Then, it will be possible to use deep learning methods such as domain adaptation or transfer learning to apply these techniques to other microscope setups or modalities without multiview capabilities. This would result in better imaging quality and 3D resolution which will be able to improve segmentation, tracking, fewer artifacts and expanding the potential for biological imaging across different platforms and experimental setups.
As the first project, Fuse My Cells is also based on points of interest:
- Open source + FAIR (Findable, Accessible, Interoperable, Reusable)
- Supervised learning, it involves annotated datasets to maintain control over performances.
- In silico annotations, a computer labeling method to avoid manual annotation and its drawbacks.
- 3D image-to-image analysis tasks, an image analysis tasks which aim to predict an output image from the input image by training a deep learning algorithm on a database built for this task.
- 3D image-to-image fusion/restauration (using deep learning)
- 3D lightsheet microscopy images
For any questions, please contact Dorian Kauffmann: dorian.kauffmann@france-bioimaging.org.