Automating fluorescence microscopy: the Roboscope project
Being designed in response to imaging challenges, the Roboscope is the product of a collaboration between Marc Tramier’s team (FBI Bretagne-Loire node) with Julia Bonnet-Gélébart, research engineer, Jacques Pécréaux’s team of the Institut Génétique & Développement de Rennes (IGDR), and the Inscoper company, spin-off of the lab. This technology could become a great timesaver for fluorescence microscopy.
Allowing the automation of fluorescence microscope acquisitions, the Roboscope is an embedded technology based on a deep learning algorithm. To be precise, it is a predesigned event-driven acquisition (PEDA) based on a learning automatization of any cellular changes tracked by fluorescence. Catching rare and fast cellular events then becomes possible!
The use of the Roboscope would also save precious time of research, providing users with results without the need to stand by the microscope during acquisition. This technology goes beyond as they will be able to recover the data already classified and with only the specific points of illumination that they have previously triggered.
A broad range of applications
The teams have almost finished to develop an entire algorithm monitoring the cell cycle progression in mitosis. These events specific to the cellular division correspond to major challenges in the control and treatment of cancer progression (Kops, 2005). As the cell cycle study is needed to understand several biological processes helping the development of targeted drugs, the technology aims to monitor efficiently and automatically simple cell models through their division cycle.
And this is not its only benefit: this automatized fluorescence microscopy acquisition can be adapted in very diverse fields. From a cell cycle progression analysis to specific analysis, organelles, proteins and biological events can be tracked or activated within cells. A noteworthy advantage of the integrated device that – we hope – will be deployed widely in the future.
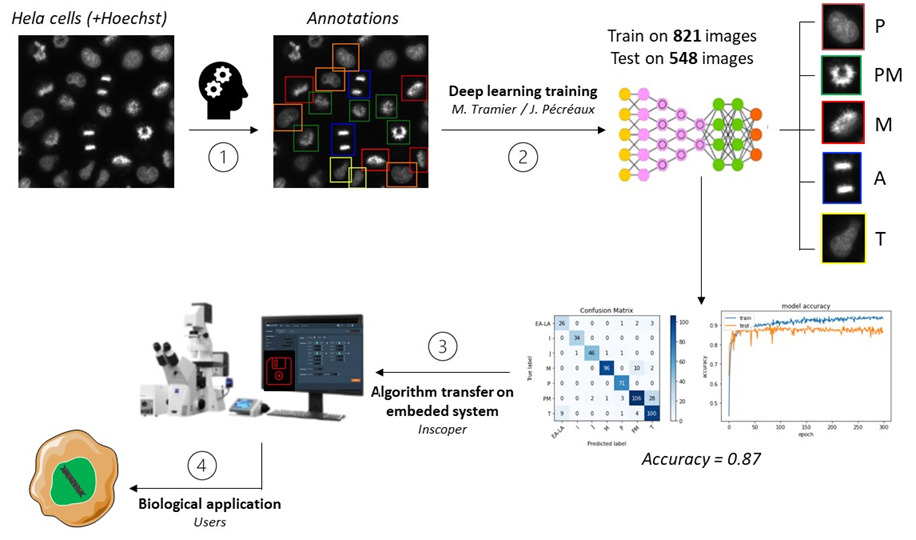